Climatological
Probabilities
A new feature has been added to the
standardized anomaly maps. The color filled regions on the maps are the
maximum
climatological probability of
the forecast meteorological
parameter reaching the predicted standardized anomaly. This is based
solely on the 60 year climate data set. This is not a
probabilistic forecast, nor is it a tool to derive a
"one in x number of year" event.
The climatological probabilities
are derived using the Chebyshev
Inequality. This is a
probability theorem which states that in
any data sample or probability distribution, nearly all the values are
close to the mean value and provides a quantitative description of
"nearly all" and "close to".
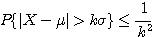
σ =
standard deviation
k =
standardized anomaly
μ =
mean
X =
random variable (height, temperature or other meteorological parameter)
In other words, no more than 1/k2
of the values are more than k
standard deviations ( k is
the value of the standardized anomaly) away from μ (the
mean).
Example:
If the GFS suggests a 120 hour
forecast of 500 hPa
Heights at -6.0 standard deviations, we can compute this climatological
probability using a "poor man's" PDF. This is done by taking 1 over the
anomaly value, squaring it, multiplying the squared value by 2 (to
account for both sides of the median), than converting to a percent. So
1/62 =
1/36, 2*36 =72, and 1/72 = 0.0138 or ~1.4%
(see color bar on graphic).
In other words, the probability that
the 500 hPa heights will differ
from the climatological mean by more than 6 standard deviations is
<= to 1.4%. Likewise the probability that the 500 hPa height will
lie within 6 standard deviations of the mean is >= 98.6% . Please be
mindful that we are using model forecast data and the various problems
and biases of each model.
Since meteorological data does not
typical fall in line with a Gaussian
distribution, the ideal frame of reference for the standardized
anomalies is a PDF for a given meteorological parameter on the grid.
But since we can not easily compute a PDF for all of the points we
forecast for, we opt for computing these climatological probabilities
using this method. This accounts for the climatological variance
and
provides a more reasonable climatological probability than extracting
sigma values from a standard Bell Curve.